Where is DevOps in 2024?
Share on socials
Where is DevOps in 2024?
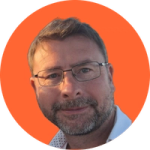
Jody Cox
24th July, 2024
18 min read
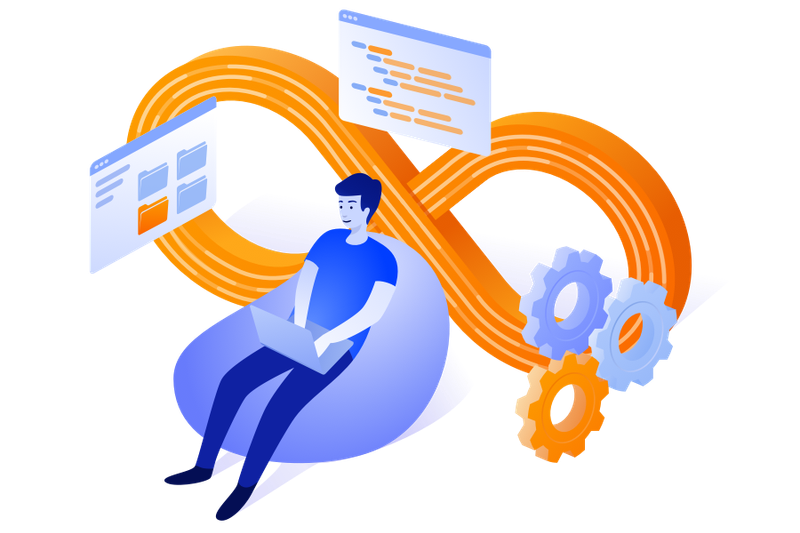
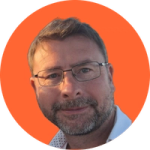
Jody Cox
24th July, 2024
18 min read
In 2024, DevOps is undergoing a significant transformation driven by artificial intelligence (AI) and machine learning (ML) advances. Intelligent applications and platforms are reshaping the software development lifecycle (SDLC), enhancing productivity, and streamlining operations. Today we will explore the rise of intelligent developer platforms, the integration of AI in platform engineering, the platform-as-a-product strategy, and the benefits and challenges of AI-enabled DevOps. Additionally, we will discuss the impact of new EU cyber regulations on DevSecOps practices, the evolving role of methodologies like Impact Engineering, and the challenges posed by DevOps tooling sprawl.
Introduction
DevOps, a set of practices that combines software development (Dev) and IT operations (Ops), aims to shorten the development lifecycle and deliver high-quality software continuously. Over the past decade, DevOps has evolved significantly, with automation and AI playing crucial roles in this transformation. As we move further into 2024, the integration of AI in DevOps is not just a trend but a necessity for organisations aiming to stay competitive in the fast-paced digital landscape.
What's been happening so far in 2024
The rise of intelligent developer platforms
Let's kick things off with one of the most exciting developments: the rise of intelligent developer platforms. From what we have seen, internal developer portals (IDPs) were heavily piloted from 2022 to 2024 in an attempt to improve the developer experience. These portals serve as interfaces for developers to access internal platform capabilities, reducing cognitive load and providing golden paths for various technologies. A raft of new products is leading the charge, offering cloud-native platform builders that streamline the developer experience and reduce complexity. We at The Adaptavist Group have developed a challenger platform called Venue.
IDPs are becoming the backbone of digital transformation, providing self-service capabilities that improve efficiency, reliability, adaptability, and cost reduction. By leveraging AI, these platforms can automate tasks, generate documentation, analyse code for errors, and even suggest code improvements based on inputs. Organisations are increasingly adopting the platform-as-a-product strategy, where a digital platform serves as the core product, enabling collaboration, innovation, and value creation within an ecosystem. This approach focuses on facilitating interactions between users, developers, and partners, creating network effects, and driving growth.
AI-augmented SDLC in platform engineering
Next, let's discuss how AI is revolutionising the software development lifecycle (SDLC). AI and ML are increasingly integrated into the SDLC, from planning and designing to development, testing, deployment, and maintenance. AI-powered tools like GitHub’s Copilot, GitLab Duo, and OpenAI's models are revolutionising code generation, optimisation, and error analysis. AI-driven platforms enhance infrastructure management, data integration, and personalised developer experiences, making the development process more efficient and effective.
For instance, AI-augmented platforms can optimise computing, storage, and network resources, enabling organisations to fine-tune operations and enhance performance. AI-driven data integration and analysis streamline data preparation and management, introducing agility and efficiency in handling extensive datasets. The introduction of AI copilots has already begun transforming the build phase of continuous integration (CI) by assisting developers with code creation and auto-completion. However, the continuous delivery (CD) phase remains challenging due to regulatory and compliance issues that slow delivery. Intelligent DevOps aims to address these challenges by increasing automation while maintaining compliance, security, and quality.
It seems logical to switch from multiple-point solutions to DevOps platforms to streamline application delivery. This will enable a shift to the growing importance of intelligent DevOps in accelerating time to market and improving the software development cycle and developer experience.
Planning and testing with AI
Thanks to AI, planning and testing are getting a major upgrade. The next generation of AI tools is expected to revolutionise these phases of the SDLC. Intelligent DevOps can help teams ensure goal alignment, analyse impacts, and provide accurate estimates for development efforts. AI can also enrich requirements and write tests, enabling test-driven development and reducing the need for expensive test developers.
AI-augmented testing can identify defects earlier in the process, significantly reducing the costs associated with downtime or security breaches. For example, AI-driven test generation can automate the creation of test cases, ensuring comprehensive coverage and improving the reliability of software releases. By focusing on doing more with less, organisations can achieve their goals within the framework of regulatory requirements and business constraints.
Security concerns and AI in DevOps
Security is always a hot topic, and it's even more critical with the advent of AI in DevOps. Recent research has demonstrated the potential of autonomous AI systems to identify and exploit security vulnerabilities. For example, researchers have used GPT-4 to autonomously hack zero-day vulnerabilities, highlighting the need for robust security measures in AI-augmented DevOps. To mitigate security risks, organisations must build solid monitoring systems, manage security problems proactively, and continuously refine source data. AI models should be trained according to new best practices and business needs, and critical processes should not be entirely managed by AI without human supervision.
The European Union has introduced new cyber regulations aimed at enhancing cybersecurity across member states. These regulations, such as the EU Cybersecurity Act and the General Data Protection Regulation (GDPR), impose stringent requirements on organisations to protect data and ensure digital infrastructure security. As a result, DevSecOps practices are becoming increasingly important to comply with these regulations and mitigate security risks.
DevSecOps integrates security practices into the DevOps process, ensuring that security is considered at every stage of the SDLC. The new EU cyber regulations require organisations to implement robust security measures, conduct regular security assessments, and ensure compliance with data protection standards. AI can play a crucial role in enhancing DevSecOps by automating security testing, identifying vulnerabilities, and providing real-time threat detection and response.
For example, AI-driven security tools can analyse code for potential vulnerabilities, monitor network traffic for suspicious activity, and generate alerts for security incidents. By integrating these tools into the DevSecOps pipeline, organisations can proactively address security issues and ensure compliance with EU regulations. This not only enhances the security of their digital infrastructure but also builds trust with customers and stakeholders.
DevOps tooling sprawl
One significant challenge in the DevOps landscape is tooling sprawl. As organisations adopt DevOps practices, they often find themselves using a myriad of tools for different stages of the SDLC. The toolchain can become overwhelmingly complex, from CI/CD pipelines and version control systems to monitoring and security tools.
Tooling sprawl can lead to several issues. Firstly, it increases the cognitive load on developers and operations teams, who need to learn and manage multiple tools. This can slow down development processes and reduce productivity. Secondly, integrating various tools can be challenging, leading to fragmented workflows and data silos. This fragmentation can hinder collaboration and make gaining a holistic view of the development process difficult.
Moreover, managing a sprawling toolchain can be costly. Licensing fees, maintenance costs, and the need for specialised skills to manage different tools can add up, straining budgets. Additionally, the lack of standardisation can lead to inconsistencies in processes and outputs, affecting the quality and reliability of software releases.
To address tooling sprawl, organisations need to adopt a more strategic approach to tool selection and integration. One solution is to use integrated DevOps platforms that offer a comprehensive suite of tools within a single ecosystem. These platforms can streamline workflows, reduce cognitive load, and provide a unified interface for managing the entire SDLC.
Another approach is to adopt open standards and APIs that facilitate seamless integration between different tools. By ensuring that tools can communicate and share data effectively, organisations can create a more cohesive and efficient DevOps environment. Additionally, adopting a platform-as-a-product strategy can help centralise and standardise tool usage, reducing sprawl and improving manageability.
Organisations should also prioritise regular audits of their toolchains to identify redundancies and inefficiencies. By periodically reviewing and rationalising their tools, they can ensure they use the most effective and necessary tools for their specific needs.
Benefits and challenges of AI-enabled platform engineering
Integrating AI in platform engineering offers numerous benefits, including enhanced decision-making, real-time monitoring, cost reduction, and improved developer support. AI-augmented platforms analyse customer feedback, usage data, and other relevant sources to provide accurate, data-driven insights. This enables businesses to make informed decisions and prioritise development efforts effectively. These platforms offer real-time insights and performance monitoring, suggesting improvements and automating routine tasks. This proactive approach helps identify and resolve issues before they impact users.
By automating tasks like data analysis and product testing, AI-enabled platforms reduce the need for human resources, cutting costs. This is particularly beneficial for organisations facing budget constraints or talent shortages. AI enhances discoverability and efficiency, supporting developers, platform engineers, and managers. This leads to a more streamlined development process and better collaboration across teams.
However, there are also challenges associated with implementing AI in platform engineering. Security risks are a significant concern, as AI systems can introduce vulnerabilities, especially if the training data contains flawed or outdated code. Continuous monitoring and updating are essential to mitigate these risks. For example, AI-generated code may inadvertently introduce security flaws that malicious actors could exploit. The accuracy of AI-generated content depends on the quality of the training data. Poor data can lead to unreliable outputs, necessitating human oversight and intervention. Organisations must invest in high-quality data and robust validation processes to ensure reliable AI outputs. Implementing and maintaining AI systems can be complex, requiring specialised skills and resources. Organisations must be prepared to invest in training and development to build the necessary expertise within their teams.
The platform-as-a-product strategy
The platform-as-a-product strategy is gaining traction as businesses recognise the value of building developer platforms that serve as core products. This approach focuses on creating ecosystems where application engineering teams can access tools, governance, and support from platform engineering teams, fostering collaboration, innovation, and value creation. By providing a foundation for interactions and transactions, platforms can generate network effects, where the platform's value increases as more participants join.
A developer platform typically includes a suite of tools and services that facilitate application development, deployment, and management. These platforms are designed to reduce the cognitive load on application engineers, standardise processes, and ensure compliance with organisational policies and industry regulations.
Platform engineering teams play a crucial role in this strategy by building and maintaining the developer platform, providing governance, and supporting application engineering teams. Their goal is to create a seamless and efficient development environment that empowers developers to focus on innovation and delivering value.
For instance, a developer platform might include integrated CI/CD pipelines, version control systems, monitoring and logging tools, and security services. By centralising these tools within a single platform, organisations can streamline workflows, reduce complexity, and improve collaboration between teams.
The platform-as-a-product strategy also emphasises the importance of user experience. Just like any other product, the developer platform should be user-friendly, reliable, and continuously improved based on user feedback. This approach ensures the platform remains relevant and valuable to its users, driving adoption and fostering a culture of innovation.
By adopting the platform-as-a-product strategy, organisations can increase innovation, scalability, and flexibility. Platforms encourage third-party development, resulting in diverse applications and continuous improvement. This fosters a culture of innovation and allows businesses to tap into the collective intelligence of their ecosystems.
Platforms also offer scalability by allowing businesses to expand offerings rapidly and adapt to changing market dynamics. This scalability is crucial for organisations looking to grow and stay competitive in a fast-paced digital landscape.
Additionally, platforms can generate new revenue streams through transaction fees, licensing, subscriptions, and advertising. By leveraging the ecosystem's growth, businesses can tap into previously untapped revenue streams.
To implement a platform-as-a-product strategy, organisations should start by identifying the core tools and services that will serve as the foundation for the developer platform. This could include existing tools or new offerings designed specifically for the platform. Aligning the platform with business goals and target markets is essential for success.
Next, organisations should define the ecosystem by identifying key stakeholders and partners who will participate in the platform. Building a strong ecosystem is essential for the platform's success, as it relies on the contributions and interactions of its participants. The platform should also have its own product owner to manage the iterations and feedback.
Fostering collaboration is also crucial and central to the product owner's role. Providing tools, resources, and support to encourage development and integration can create a vibrant developer community. This community can drive innovation and continuous improvement, ensuring the platform remains relevant and valuable.
Continuous evolution is another key aspect of the platform-as-a-product strategy. Regularly gathering feedback from developers and partners can help improve work and productivity. This iterative approach ensures the platform evolves to meet the changing needs of its ecosystem. As with all things in engineering, nothing ever stands still.
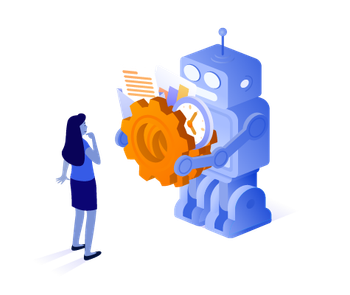
What's next in Automated Software Delivery (AutoSD) - Emerging Trends
Join experts at this fireside chat as they explore the cutting-edge trends shaping the future of Automated Software Delivery (AutoSD), including AI and AIOps, DevEx, and self-serve, and cloud development environments.
Future outlook
Looking ahead, the future of DevOps lies in further integration of AI and ML. Predictions indicate that by 2027, 75% of organisations will have switched from multiple-point solutions to DevOps platforms. Emerging technologies and practices will continue to shape the DevOps landscape, emphasising the importance of staying ahead in this dynamic field.
Intelligent DevOps will enable businesses to increase automation for improved efficiency while maintaining compliance, security, and quality. By focusing on doing more with less, organisations can achieve their goals within regulatory requirements and business constraints. Integrating AI in DevOps is transforming the software development lifecycle, offering numerous benefits while also presenting challenges. Organisations must navigate these complexities to leverage AI's full potential, enhancing productivity, reducing costs, and improving the developer experience.
Businesses that embrace these trends and invest in AI-driven DevOps platforms will be better positioned to innovate, scale, and compete in the digital age. By staying informed and adaptable, organisations can harness the power of AI-enabled DevOps to drive growth and success.
Conclusion
The integration of AI in DevOps is transforming the software development lifecycle once again, offering numerous benefits while also presenting challenges. Organisations must navigate these complexities to leverage AI's full potential, enhancing productivity, reducing costs, and improving the developer experience. As DevOps continues to evolve, staying informed and adaptable will be key to success.
Ready to revolutionise your DevOps strategy and stay ahead of the trends?
Connect with our experts at Adaptavist to discuss your DevOps needs and explore how AI-driven solutions can transform your software development lifecycle. Don’t get left behind—embrace the future of intelligent DevOps today!
Get in touch today to talk to our experts.
Written by
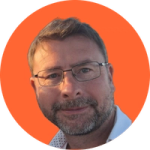
Jody Cox
Principal Sales Executive
Jody leverages over 19 year's agile expertise and nearly a decade in cloud and DevOps to design comprehensive agile delivery approaches and guide clients through digital transformation.